๐ง How to Extract Insight from Anything (3 Tips)
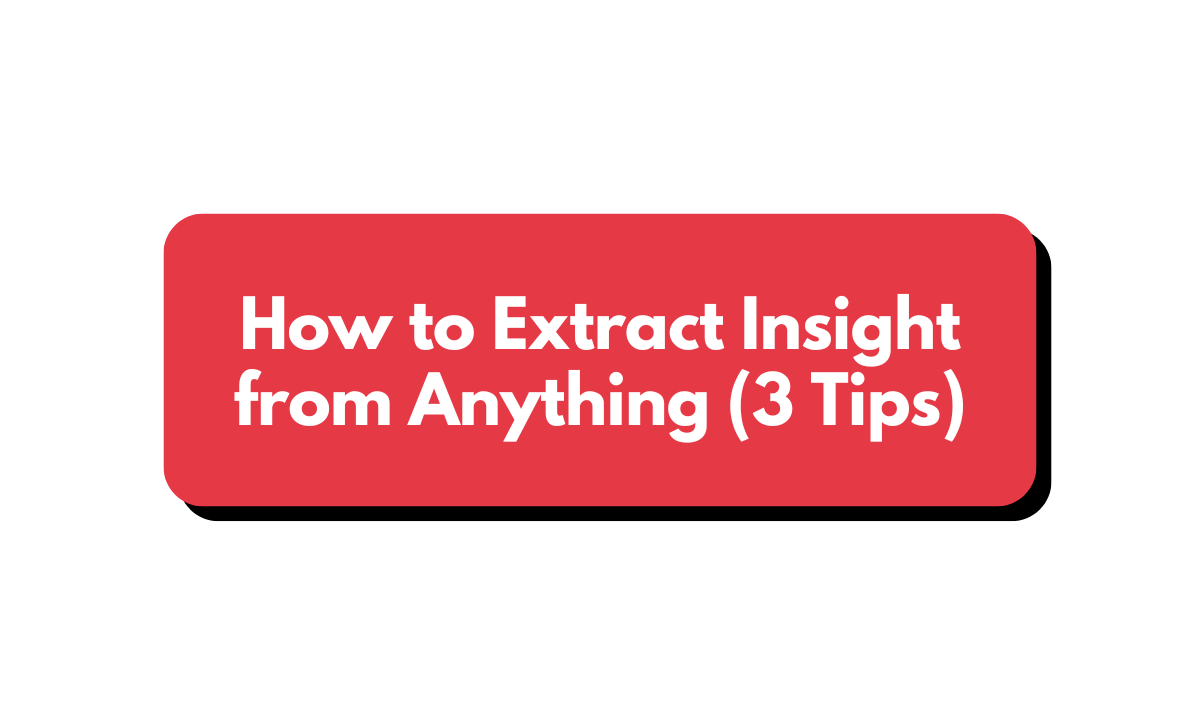
One of the struggles early on in my career (I worked in the data analytics space) was to come up with proper "insight" in my analyses.
I struggled for a couple reasons:
- I wasn't sure what counted as "insight." (What if I'm pointing out something that's obvious to everyone?)
- I didn't have a systematic approach. Sure, I'd try to copy good hypotheses from past projects and replicate. But I'd struggle when I dealt with a new client or new industry.
Years later, I have a much better understanding of what actually qualifies as insight. I also have a much more replicable approach to uncovering it.
So without further ado, here are 3 foolproof ways to uncover insights from anything:
#1 Find where the pattern "breaks."
There's no insight when something happens as expected. But think about what the corollary of this suggests. It tells us that insight occurs when something unexpected happens.
And thus when you're on the quest for insight, one of the most useful things you can ask becomes:
Where does the pattern break?
Sometimes this is simple and easy to identify. For instance:
- A sudden dip after consecutive quarters of steady growth
- A market that is underperforming the rest of the region
- A metric that is negative when most other ones are all positive
But sometimes this is less obvious. And this is where intentionally looking for pattern breakers can help you uncover what others fail to see. That's when you deliver insight.
Here's an example: Monthly sales appear to be steady over the past 12 months. No surprises (and no particular insight) so far.
However, when you analyze the sales composition, you suddenly realize the top-selling categories have completely changed in the past month:
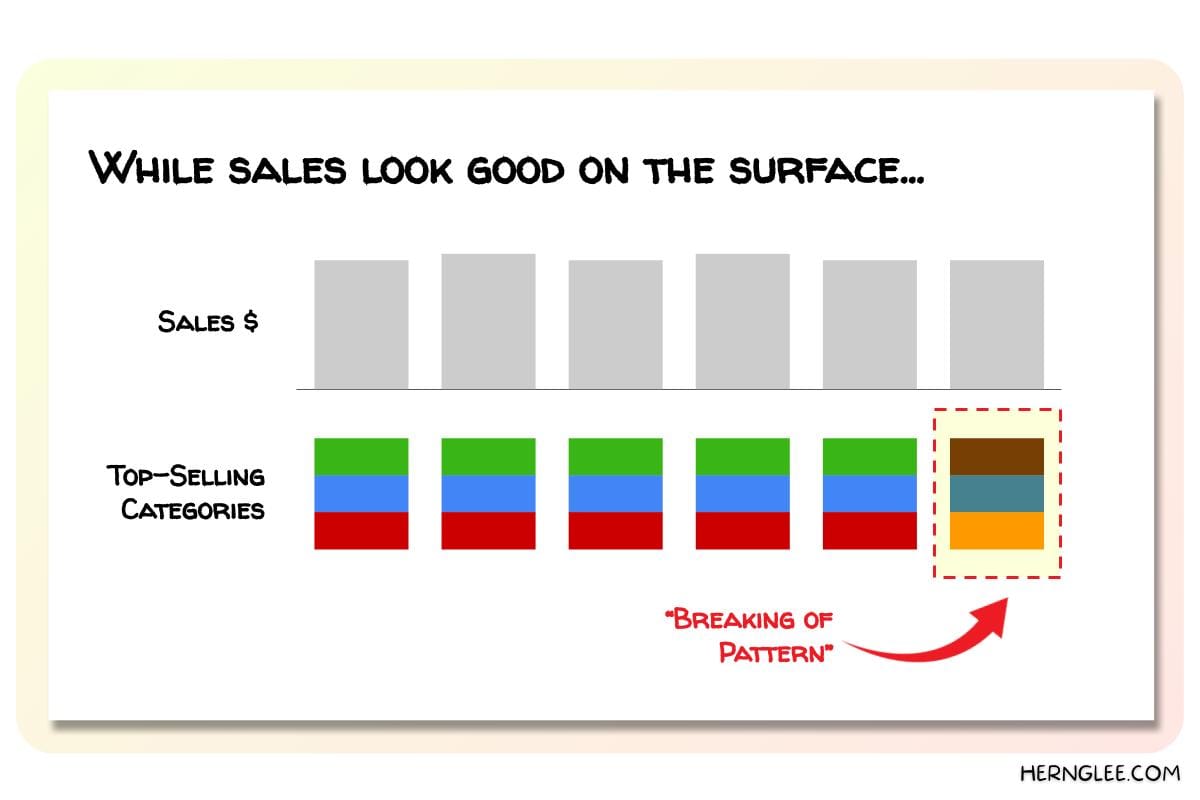
Simple observation โ but ample insight will follow.
๐ Subscribe for free to get Herng's newsletter directly in your inbox.
#2 Segment a lot (but not too much).
It turns out that most insights come from segmenting. In fact, we often encounter (and use) segmentations unknowingly all the time. For instance:
- My favorite basketball has won the last 10 games in a row (but 9 out of the 10 games were against the worst teams in the league)
- The average starting salary might have gone up over the years (but only for certain industries)
- A restaurant has extremely high ratings on Google Maps (but the 5-star reviews were all from tourists, not locals)
Segmenting is how we uncover insights that may be obfuscated by simple averages and aggregations. It is simply a process of slicing and dicing.
The key, however, is recognizing what dimensions are most useful to segment along.
As an example, let's imagine you run a lemonade stand business. Tracking sales data is simple. But insight comes from asking the following questions:
- How do my sales vary by time of day (or day of the week)?
- How do my sales vary by demographics (gender, age, etc.)?
- How do my sales vary by product type (cup size, flavor, price point)?
- How do my sales vary by customer type (first-time vs. repeat)?
When you segment rigorously, you're more likely to find instances where the pattern breaks (see Tip #1) โ and therein lies your path towards insight.
However: you cannot segment everything. And you should not segment everything.
Here's 3 reasons why.
โ ๏ธ The first is that you don't always have the right data. And there are often costs associated with obtaining that data.
For instance, to collect any type of customer demographics data:
- Does the salesperson (in a physical store) have to eyeball it and write it down, thus taking time away from serving customers?
- Do you ask your customer to fill it out (and create friction in the buying process)?
Some data dimensions are valuable and worth collecting. Some aren't.
โ ๏ธ The second is that over-segmentation often leads to noise. Even if you're sitting on a treasure trove of granular data, you need to ensure integrity in your segmentation efforts.
For instance, let's go back to our lemonade stand. Imagine if you wanted to analyze the sales patterns of male customers between the ages of 35-40 who visit on weekdays during lunch hours.
Sure, you'll get more than enough dimensions to analyze.
(But you might also end up extrapolating from a sample size of 2 people.)
โ ๏ธ The third and last thing is that there isn't always actionability.
(But there is almost always a cost to extracting, interpreting, validating, and revalidating the data.)
More data isn't always better. Sometimes it's just a distraction. Especially when there's no actionability.
For instance: let's say we somehow managed to identify that men in suits tend to buy lemonade more often than men in shorts.
(But so what? What can we actually action on based on this seemingly insightful nugget?)
Segmentation is important and helpful. But don't overdo it.
#3 Define the benchmark (and spot the gap).
What if there's no obvious break in pattern, and you've run out of dimensions to segment?
That's where redefining the benchmark comes in.
Remember that in Tip #1, we established that "insight occurs when something unexpected happens."
But someone needs to establish what that expected baseline is!
In some instances, that's easy.
For instance, we might just look backwards: e.g. my lemonade stand brought in $50 a day on average last year, so that's probably what I can expect this year.
However, in some instances, the benchmark is less clear. It might even be erroneous.
For example, imagine that I run a chain of fast-food restaurants, and I operate across 100 locations. And I'm about to open up my 101st store next month.
Question: What revenue can I expect to generate for my new store?
Well, I could look at the average sales for the other 100 stores ($10K/month) and call it a day. So maybe I should expect $10K/month?
But it turns out half of my 100 existing stores are next to metro stations with heavy footfall (while my new store isn't). And the average sales for those 50 other non-metro stores is only $10K/month.
So maybe I should expect only $10K/month?
But wait โ I now remember that only 10 of those 50 stores were renovated in the last year, while the other 40 are old and run down. My new store is obviously renovated, so...
...you get the picture. This is where defining the proper benchmark brings incredible insight and critical value.
Once you realize this, you'll see opportunities to define (and sometimes redefine) the right benchmark everywhere. You'll learn to ask questions like:
"How high is high?"
Or, you might also ask:
"What does 'good' look like?"
That is the only way you can establish whether something is good, bad, or meh. For instance, imagine the following statements:
- Your revenue is growing at 50% year-over-year
- Your product penetration in a given market is at 5%
- You secured 20% of your clientโs annual budget
Which headlines call for celebration? And which ones are of concern?
People have no idea, unless you help benchmark and contextualize.
Then you might realize 50% growth is pathetic (market is growing at 100%!), and 5% penetration is actually very commendable (since your previous products all plateaued at 3%).
And that's insight.
* For a refresher on data vs. insight, I cover this from pages 6 to 10 in my StratOps playbook: